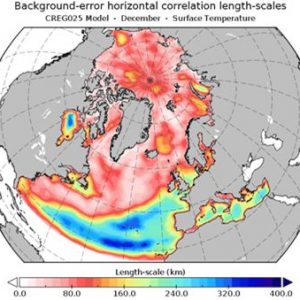
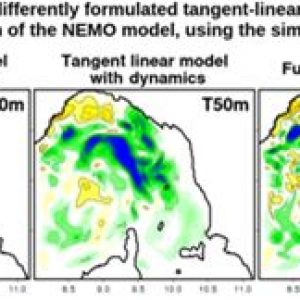
Variational Data Assimilation System
ISMAR has developed a cutting-edge variational data assimilation framework to support a range of applications, from algorithmic advancements to climate reconstructions and the validation of emerging observational data. This system employs a hybrid covariance scheme and a simplified four-dimensional data assimilation process, optimizing in situ and remote observations alongside prior numerical forecasts to deliver precise analyses of ocean states for improved forecasting and climate reconstructions.
The core of ISMAR’s assimilation system, initially known as OceanVar, efficiently models horizontal and vertical covariances using recursive filters and multivariate empirical orthogonal functions, respectively. OceanVar also integrates support for hybrid ensemble-variational covariances and offers both strong and weak constraint four-dimensional assimilation, achieved through simplified tangent-linear and adjoint versions of the NEMO ocean model.
In addition to multivariate balance control, OceanVar features robust options for nonlinear quality control of observations and detailed representation of model error. This system is actively used for reconstructing historical ocean climates, conducting coupled data assimilation experiments, and testing novel satellite data acquisition algorithms for both current and future observational missions.
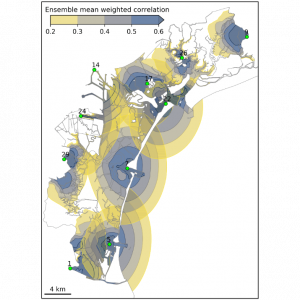
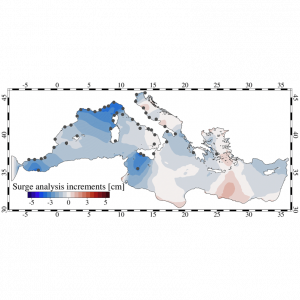
Sequential Data Assimilation System
To support applications from marine forecasting to the climatological reconstruction of marine variables, ISMAR has developed a sequential data assimilation system grounded in the Kalman filter, with perturbations generated for forcings, boundary conditions, and physical states simulated by a finite element hydrodynamic model.
ISMAR’s system utilizes Ensemble Kalman Filter (EnKF) techniques. It creates an ensemble of states within the hydrodynamic system, allowing calculation of variances and covariances among dynamic variables to accurately specify the evolving background error matrix. Data assimilation with the Kalman filter is sequential, integrating observations immediately as they are recorded. The ensemble generation employs routines capable of producing pseudo-Gaussian spatial perturbations that can be applied to initial states, forcings, and boundary conditions. Depending on the physical system being modeled, users can adjust ensemble size and apply localization techniques with an appropriate spatial decorrelation scale.
The ensemble data assimilation system is used in conjunction with the SHYFEM finite element hydrodynamic model, also developed at ISMAR. Studies have focused on reanalysis and forecasts, primarily aimed at reproducing sea level variations.
References:
(Bajo et al., 2019, 2023), Ferrarin et al. (2021)
Bajo, M., Ferrarin, C., Umgiesser, G., Bonometto, A., & Coraci, E. (2023). Modelling the barotropic sea level in the Mediterranean Sea using data assimilation. Ocean Science, 19(3), 559–579. https://doi.org/10.5194/os-19-559-2023
Bajo, M., Međugorac, I., Umgiesser, G., & Orlić, M. (2019). Storm surge and seiche modelling in the Adriatic Sea and the impact of data assimilation. Quarterly Journal of the Royal Meteorological Society, 145(722), 2070–2084. https://doi.org/10.1002/qj.3544
Ferrarin, C., Bajo, M., and Umgiesser, G.: Model-driven optimization of coastal sea observatories through data assimilation in a finite element hydrodynamic model (SHYFEM v. 7_5_65), Geosci. Model Dev., 14, 645–659, https://doi.org/10.5194/gmd-14-645-2021, 2021.
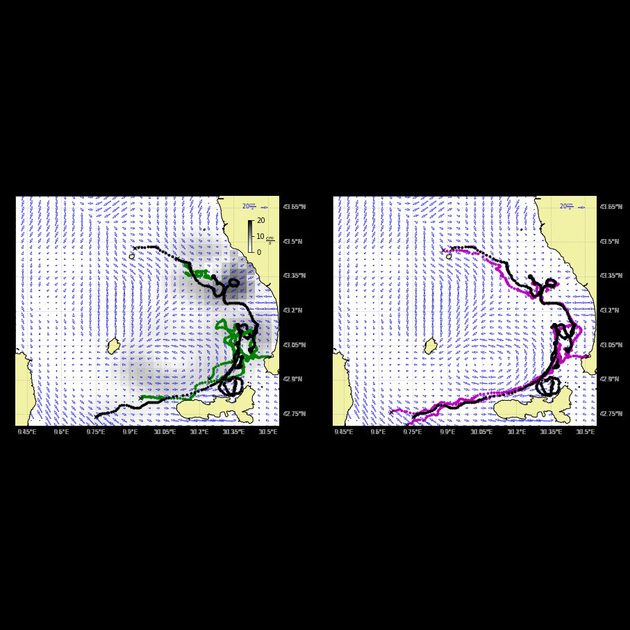
LAVA (LAgrangian Variational Analysis)
LAVA is an open-source software developed for the integration of diverse oceanographic data—a process known as “data fusion” or “data blending”—which is especially valuable for managing marine emergencies such as oil spills or search and rescue missions.
Developed by CNR-ISMAR in collaboration with the University of Toulon and the University of Miami, LAVA provides optimal fusion of current velocity and direction information from multiple oceanographic instruments. Using a Lagrangian and variational approach, LAVA minimizes the distance between observed Lagrangian positions and those computed by numerically integrating an Eulerian field. The figure below shows a specific example of LAVA applied to radar-measured velocities.
In the left panel, trajectories derived from original radar velocity data (green) diverge from those observed by drifters (black). By incorporating additional drifter data from the surrounding area and applying LAVA, the velocities are locally adjusted, bringing the new trajectories (purple, right panel) closer to the observed drifter paths. This improvement is significant for practical applications, as the more accurate trajectory estimates produced by LAVA substantially reduce the uncertainty radius for search operations and oil spill containment efforts.